Event »Model of a fair data economy in Germany and Europe«
During the event, our project FAIRDataSpaces will present its first demonstrators, discuss win-win for science and industry as well as architectures for data exchange across data spaces with the participants.
In addition to keynotes by Bettina Stark-Watzinger (Federal Minister of Education and Research), Prof. Reimund Neugebauer (President of the Fraunhofer-Gesellschaft), Prof. York Sure-Vetter (Director of the National Research Data Infrastructure) and Iris Plöger (Member of the BDI Executive Board), Prof. Irene Bertschek (ZEW) as well as keynote speeches by many other experts, we are looking forward to a lively exchange!
What you can expect:
- Contributions on the way to a fair data economy
- the draft of a future framework of action for science, politics and industry
- Impulses for research perspectives on the data economy
- Deep-dive sessions (more detailed info and abstracts at the bottom of this page)
- Live demonstrators
Click here for more information: https://www.iuk.fraunhofer.de/de/events/fair-data.html
Programme of the event
9:30 |
Prof. Reimund Neugebauer |
9:45 |
Bettina Stark-Watzinger |
9:55 |
Prof. Boris Otto |
10:05 |
Impulse: Forschungsperspektiven der Datenökonomie
|
10:35 | KAFFEEPAUSE |
10:45 |
Panel-Diskussion zum datenökonomischen Leitbild
|
11:45 |
Tandem-Impulse: Einführung in die Deep-Dive-Sessions
|
12:30 | MITTAGSPAUSE |
13:15 |
Deep-Dive-Sessions
|
14:30 | KAFFEEPAUSE |
14:40 |
Prof. Boris Otto Zusammenfassung der Ergebnisse und Ausblick |
15:00 | ENDE DER HAUPTVERANSTALTUNG |
15:00 |
Fortsetzung der FAIR Data Spaces Sessions
|
* Die ersten drei Deep-Dive-Sessions sind Bestandteil des zweiten Anwenderworkshops des BMBF-Projekts »FAIR Data Spaces« und werden ab 15 Uhr in vertiefenden Sitzungen bis ca. 17 Uhr fortgesetzt. |
Programme and Abstracts Deep Dive Sessions
- Win-win for science and industry through FAIR Data Spaces
- Architectural foundations for data exchange across data spaces
- Demonstrators for data exchange between industry and science
Following the official program of the event, these three deep dives will go into a second round from 3pm to approximately 5pm to further explore the topics and discussions from the first sessions (1:15pm – 2:30pm). Below is a brief description of the three deep dive sessions of the FAIR Data Spaces project.
Deep Dive: Win-win for science and industry through FAIR Data Spaces
The data exchange between science and industry offers the opportunity to generate added value for both sides. For a successful collaboration between both domains, it is first necessary to create a shared vision that enables the creation of an infrastructure for data provision and trustworthy data use.
The “FAIR Data Spaces” project creates a roadmap with visions and goals for a collaboration between science and industry and builds a common community. In addition to considering legal and ethical aspects, this serves as the basis for technical building blocks and practical implementation, as seen in the parallel deep-dives.
In the deep dive “Win-win for science and industry through data sharing”, we first show how the FAIR Data principles affect industry beyond research – complemented by an impulse from the perspective of the legal framework that is significant for industry. Building on these foundations, we will explore in a joint, interactive brainstorming session the question of how a shared community can be used to achieve benefits for both sides.
13:15 – 14:30 h | Community Building |
13:15 – 13:25 h | Introduction FAIR Data Spaces |
13:25 – 13:40 h | Impulse: FAIR and FRAND (EU Data Act) |
13:40 – 13:50 h | Community Poll |
13:50 – 14:25 h | Community Brainstorming & Discussion |
14:25 – 14:30 h | Wrap-up and Preview Session 2 |
15:00 – 17:00 h | Roadmap For A Common Community |
15:00 – 15:10 h | Summary of Session Community Building |
15:10 – 16:10 h | Community Brainstorming and Diskussion –
Results & Conclusions |
16:10 – 16:20 h | Break |
16:20 – 16:55 h | Roadmap Draft |
16:55 – 17:00 h | Wrap-up |
Deep Dive: Architectural foundations for data exchange across data spaces
This session will start with a panel discussion of three experts on data spaces with different professional backgrounds. The panelists are Lars Nagel, CEO at the International Data Spaces Association, Sebastian Kleff, Co-Founder and CEO at Sovity, and Klaus Ottradovetz, VP Global Service Delivery at Atos. After this round of experts, the discussion will be opened to all session participants.
Deep Dive: Demonstrators for data exchange between industry and science
This deep dive will be all about demonstrators. Demonstrators are used to prove the feasibility of concepts developed within the project. Participants had the opportunity to choose from three demonstrators – the NFDI4Biodiversity Demonstrator, the FAIR Data Quality Assurance and Workflows Demonstrator, and the FAIR Cross-Platform Data Analysis Demonstrator.
Demonstrator AP 4.1
Deep Dive Title: FAIR-DS Demonstrator NFDI4Biodiversity
Demonstrators in FAIR-DS originate from different NFDI domains and are used as proof-of-concept showpieces for multi-cloud capabilities such as Gaia-X conformity through self-description of services. This deep dive session allows you to learn and interact with the NFDI4Biodiversity demonstrator. A key service in this project is the Geo Engine, a cloud-based research environment for spatio-temporal data processing. Geo Engine supports interactive data analysis for geodata, which includes vector and raster data. Data scientists work with geodata through exploratory analysis and achieve new insights by trying out new combinations of data and operators. Since geodata is inherently visual, the Geo Engine has a web-based user interface that supports visual analytics and provenance through an implicit definition of exploratory workflows. Results of those workflows are interactively provided, facilitating the exploratory nature of modern data science. In addition to the user interface, Geo Engine also offers a Python API, which can be used in the analytics process. As a first initial step in our FAIR Data Spaces project, we worked on a use case combining data from industry (satellite data) with data from academia (GFBio). This use case will be the basis for our session.
We will present Geo Engine, describe our initial results within the Fair Data Space project and demonstrate the developed use cases in a live coding session. In the coding session, you will first learn how to interact with the user interface of the Geo Engine. Then, we will introduce our Python API and show how to apply it to add new workflows to the Geo Engine. Finally, you will learn how components introduced via the Python API are accessible in the user interface and vice versa.
Afterwards, you will be able to use the Geo Engine yourself by bringing your own data and applying the skills you acquired during the presentation and live coding session. For this purpose, we will discuss our upload functionality and invite you to follow along at home. To participate in the “bring your own data“ section of the deep dive, please send your files in advance as instructed in the respective mail you received after registering for this deep dive. Files should be well-formatted GeoPackage, GeoJSON or CSV files. By sending us files beforehand, we can validate their compatibility in advance – this is especially helpful in case of CSV files. This way you can make the best use of your time to work with Geo Engine instead of dealing with formatting issues.
Among many preloaded data sets, Geo Engine will provide the Normalized Difference Vegetation Index (NDVI) as monthly cloud-free aggregates for Germany.
Session 1: Presentation + Live Coding (13:15 – 14:30)
13:15 – 13:35: Common Session
13:35 – 13:48: Introduce core Geo Engine concepts
13:48 – 14:05: Live Usage of Web UI
14:05 – 14:20: Live Coding Session Python API
14:20 – 14:30: Short Q&A Session
Session 2: Bring your own data (15:00 – 17:30)
15:00 – 15:10: Show import example
15:10 – 15:15: Suggest actions for data
15:15 – 17:30: Stand by for questions?
Demonstrator AP 4.2
Research Data Quality Assurance and Workflows
The breakout session will discuss the FAIR Data Spaces Demonstrator “FAIR Data Quality Assurance and Workflows” developed within FAIR Data Spaces together with NFDI4Ing. The demonstrator uses the workflow engine provided by the source code hosting platform GitLab to analyze, transform and verify research data artifacts. Within the demonstrator research data is assumed to be collected in form of CSV files by an individual researcher or a group of researchers who want to make use of features coming from the “social coding“ paradigm to maintain their research data.
The presented example workflow will include:
- Extraction of a “Frictionless Schema” from a collection of existing CSV data.
- Validation of new data based on existing schema definitions
- Assertion of data quality metrics like
- Number of missing values
- Value distribution
- Value correlations
- Generation of quality report “score cards“ for research data
- Publication of research data to repositories like Zenodo
The session is split into two parts: In the first part you will learn about the demonstrator structure and the workflow example based on a sample dataset. Together with our instructors you will be able to interactively modify a copy of the dataset and see how changes in the quality of the data set reflect in the quality report. In the second part you can bring your own data in the form of CSV files. Together with our instructors we will add your dataset into the workflow, allow validation and assertion of quality metrics. When bringing your own data please make sure that you are comfortable screen sharing for showing results or during questions with other participants and the instructors. Please avoid bringing confidential data as it very much limits the help that can be provided by the instructors. By sending us files beforehand, we can validate their compatibility in advance. Thus, you can maximize your time actually experimenting with the Demonstrator instead of dealing with formatting problems.
Session 1: Introduction & Participatory Live Coding
- Short Introduction of the Demonstrator
- Scenario
- Technical Architecture
- Introduce basic concepts of GitLab
- Repository/Files View
- Editing a file in the browser
- Workflow definition in .yml files
- Workflow visualization in traffic light system
- Basic concept of the Demonstrator
- Structure of the repository
- Where is the data?
- Where is the workflow defined?
- Workflow Pipeline steps explained
- Schema extraction
- Schema validation
- Assertion of quality metrics
- Upload of score card
- Participatory Live Coding: Examples will be on shared screen of the instructor, participants are motivated to follow along and try the things out as they are presented
- Change one of the CSV files to violate the schema
- Add a new CSV file and extract the schema
- Add a new CSV file with highly correlated data
- Structure of the repository
Session 2: Bring Your Own Data
- Short wrap up of the previous Session
- Questions?
- Insertion of “own data”: Ask one participant to share their screen and guide them through inserting their data:
- Start with forks again, (Open Question: Reuse forks or create new??)
- Remove all existing CSV files
- Dump your own data
- Other participants may follow along or simply try on their own (however during this time individual support may be limited)
- Repeat with another participant
- Ask all participants to try asynchronously with their own data
- Ask participants to share their screen with everyone if they have questions
- Timebox answering questions to 5 minutes to allow other participants to ask questions
Demonstrator AP 4.3
Cross-Platform FAIR data analysis on health data
This deep-dive/breakout session presents a Cross-Platform FAIR data analysis demonstrator. The main point about our demonstrator is to represent the process and the analysis of health-related data. For our presentation, we consider two use cases:
- Use case 1: Skin lesion classification.
Another health disease with a relevant number of patients worldwide is cancer. Skin cancer has become the most common cancer type. Research has applied image processing and analysis tools to support and improve the diagnosis process. We use the skin lesion image dataset for melanoma classification from the International Skin Imaging Collaboration (ISIC) 2019 Challenge for our image classification task. Our skin lesion image dataset consists of 33.569 dermoscopic images. Each image is classified into eight different diagnostic categories indicating the lesion types.
The official ISIC challenge training dataset and images are divided into three subsets and distributed among three stations for training. 80% of images on each Station are used as training datasets, and the other 20% are used as validation datasets. In the skin lesion use case, we illustrate incremental institutional learning in which the data is distributed across three geographically different locations.
Use_Case_Execution_Result_ISIC2019_PHT.zip
- Use case 2: Malaria outbreak analysis.
Malaria is a life-threatening disease caused by parasites transmitted to people through the bites of infected female Anopheles mosquitoes. According to the World Health Organization (WHO), there were an estimated 241 million malaria cases worldwide, with 627 thousand malaria deaths in 2020. For our analysis, we consider the malaria dataset that contains data related to the number of cases and deaths for every country from 2000 to 2017. Our dataset also provides geographical data to represent countries‘ information in world-map-based charts.
In the malaria use case, we have split the country-based information into three different subsets based on the “WHO Region” attribute available in the dataset. One data set contains data on “Eastern Mediterranean and Africa”; the other contains information on “Americas and Europe“, and the third report’s statistics for “South-East Asia, Western Pacific“. Each Station owns only one of these datasets.
Use_Case_Execution_Result_Malaria_PHT.zip
- Cross-Platform FAIR data analysis on health data
We use a Cross-platform Data Analysis infrastructure called Personal Health Train (PHT). PHT provides all the required data analysis procedures in both malaria and skin lesion classification dataset. The main elements in the PHT ecosystem are the so-called Trains and Stations. The Train encapsulates the analysis tasks using containerisation technologies. Trains contain all prerequisites to query the data, execute the algorithm, and store the results. Stations act as data providers that maintain repositories of data. A specific Train is sequentially transmitted to every Station to analyze decentralized data. The Train performs the analytical task and calculates the results (e.g. statistics) based on the locally available data.
The session is split into two parts: In the first part, you will learn about the demonstrator structure, workflow example, and executed use cases, and in the second part, you can bring your own code (Train). We will run your code on the PHT stations with our instructors. Initially, we will provide access to a GitLab repository beforehand. This repository will contain sample codes and data schemas available at each station. Participants will be invited into the GitLab repository (or they can send an access request) using their GitLab accounts to be able to apply their changes in the repository. The main branch that triggers the GitLab CI (to build and push Trains) is protected; Users create “Merge Requests“ to apply their changes.
13:15 – 14:30 Uhr | Aufbau einer gemeinsamen Community |
13:15 – 13:25 Uhr | Einführung & Vorstellung FAIR Data Spaces |
13:25 – 13:40 Uhr | Impuls: FAIR and FRAND (EU Data Act) |
13:40 – 13:50 Uhr | Community Poll |
13:50 – 14:25 Uhr | Community Brainstorming & Diskussion |
14:25 – 14:30 Uhr | Wrap-up und Ausblick Session 2 |
15:00 – 17:00 Uhr | Fahrplan für die gemeinsame Community |
15:00 – 15:10 Uhr | Zusammenfassung Session Aufbau einer Gemeinsamen Community |
15:10 – 16:10 Uhr | Community Brainstorming und Diskussion –
Ergebnisse & Fazit |
16:10 – 16:20 Uhr | Pause |
16:20 – 16:55 Uhr | Entwurf Fahrplan |
16:55 – 17:00 Uhr | Wrap-up |
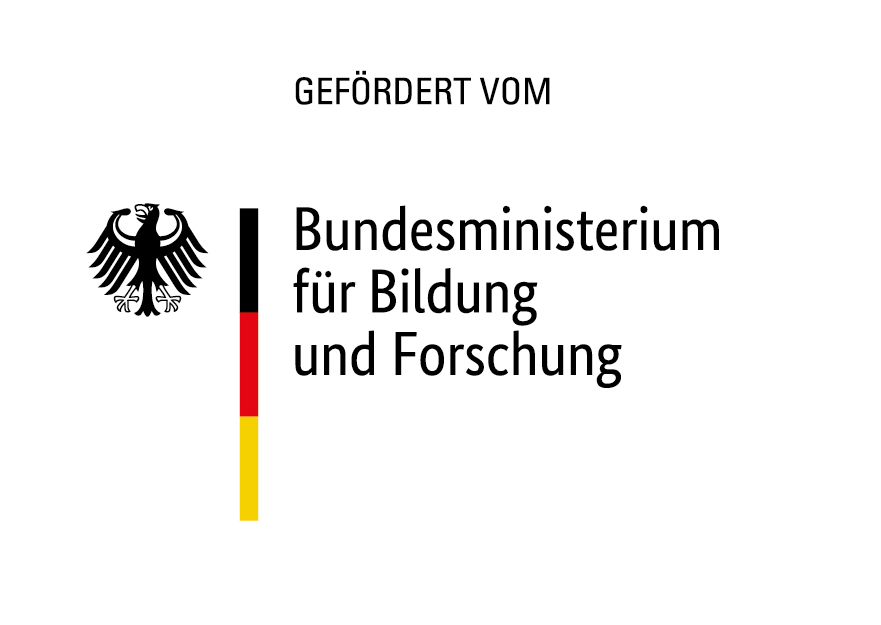