2nd FAIR Data Spaces Workshop
(held on March 15 2022)
Here you can find the post-event reports on our second FAIR Data Spaces project workshop on 15 March 2022. Our workshop was part of the BMBF event “Leitbild einer fairen Datenökonomie in Deutschland und Europa” (Model of a fair data economy in Germany and Europe), organised by the Fraunhofer-Verbund IUK-Technologie and under the patronage of the German Federal Minister of Research Bettina Stark-Watzinger. The following sessions were part of the event:
- Win-win for science and industry through FAIR Data Space
- Architectural foundations for data exchange across data spaces
- Demonstrators for data exchange between industry and science
Win-win for science and industry through FAIR Data Spaces
The deep dive “Win-win for science and industry through FAIR Data Spaces” focused on first steps towards building a common community by identifying obstacles and identifying necessary means to overcome them. An interactive setup was used to facilitate a maximum participation of the session audience.
Impulse Talk – Legal Perspective
To set the stage, the deep dive was kicked off with an introduction to the FAIR and FRAND principles. The FAIR Data Spaces project combines two different worlds: NFDI and Gaia-X. The exchange of scientific research data in NFDI is characterized by cooperative effects and by the FAIR principles (findable, accessible, interoperable, reusable). Gaia-X, on the other hand, is intended to promote the exchange of data from industry and is therefore primarily characterized by confidentiality and protectionist principles. Only in exceptional cases can an exchange of data be enforced via compulsory licenses under antitrust law (FRAND). The planned EU Data Act also plays a special role in bringing these two projects together. This provides precisely that every user should have access to data generated by him – for example, by smart devices – and should be able to share it with third parties. According to Art. 8 of the draft EU Data Act, moreover, clauses in contractual data access rights should be fair, reasonable and non-discriminatory. Thus, a bridge is built between Gaia-X and the FAIR principles for raw data without personal reference. However, there are still some legal issues that need to be addressed: For example, in the automotive sector, a reference to a person (for example, in the case of the VIN) cannot be ruled out without further ado. Also, it is not the driver of a smart vehicle who generates the data, but the electronics installed by the manufacturer. These legal questions will be discussed by ITM Münster in cooperation with FIZ Karlsruhe as part of the FAIR Data Spaces project.
The two key areas of law moving forward are intellectual property and data protection. In relation to both areas, discussants highlighted an ongoing need to establish clarity as to the applicability of current law in relation to the shared data space, as well as to the varied processing operations which may happen within the data space. Discussants also observed, however, that, in both areas of law, the legal framework is fluid and in a state of change – the EU Data Act was discussed as one element of such change. The specifics of prospective changes, however, can be dependant on factors which can be difficult to predict in advance and, accordingly, discussants observed that providing definitive interpretations of law concerning the operations of a shared data space, and the processing operations which might happen within this space, will, in relation to certain issues at least, be difficult. In this regard, discussants highlighted the need for continuous monitoring of legal developments for their impact on applicable law. Further, discussants stressed the need for further work concerning how the options offered under current law might best be utilized to legally achieve community goals concerning the shared data space. In this regard, discussants mentioned the need for translation of clarifications of applicable law, and the options the law makes available, into practice – for example via ELSA trainings.
Community Building
After this discussion on legal aspects, a short community poll to get to know the participants and their respective backgrounds was carried out, followed by a brainstorming session. During the brainstorming the participants focused on questions around data exchange and data spaces. With the help of Google Jamboard the participants were able to share their thoughts on post-its. Afterwards, the contributions to each question were discussed and concluding remarks were found. A few key remarks will be stated in the following. Given the question about examples of successful collaboration between science and industry regarding data exchange, the participants established that there is a need for connecting both domains and encouraging a close dialogue in order to aid a successful collaboration. This highlighted the importance of the FAIR Data Spaces project in connecting both domains. When asked about what the participants would like to use and/or contribute in data spaces, a certain degree of transparency to promote trust between all involved parties was emphasized. Asking about possible community stakeholders, the participants pointed out the importance of extending the community beyond science and industry to also include other domains, such as the public sector. Additionally, it was highlighted that an international positioning of the project and an alignment with related initiatives is crucial for good future prospects.
Architectural foundations for data exchange across data spaces
The deep dive on architectural foundations for data exchange across data spaces began with a panel discussion between three experts of data spaces with different backgrounds. First speaker in the round was Lars Nagel, CEO at the International Data Spaces Association, with his opening statement “One common governance for data spaces – From application to legal frameworks”. He was followed by Sebastian Kleff, Co-Founder & CEO at Sovity, who presented on “The technical implementations and their evolutions – One joint goal of data sovereignty”. Last but not least, Klaus Ottradovetz, VP Global Service Delivery at Atos discussed “One holistic trust framework of data economy – Federated concepts enable trust”. The panel discussion revealed some very important “cornerstones” for enabling data exchange across data spaces:
- Fair data economy in the European market
- Trust established by technology
- Decentralization and federation
- Automated utilization of data
- Full sovereignty in data sharing
- Autonomous and sovereign data processing
In the second part of the session attendees discussed points that came up during the panel discussion, like the big variety of initiatives and stakeholders, and the usability of the solutions. Also, a deeper insight to the actual technical situation was given and discussed. A big question of the audience was, how to make the solutions usable in an easy way, which could not be answered conclusively yet at the moment. In the end the great majority agreed that a project such as FAIR Data Spaces needs decades to come to success and that the change in technology comes along with change in culture and collaboration only. A recording of the deep dive session can be found here.
Demonstrators for data exchange between industry and science
This deep dive was all about demonstrators. Demonstrators in FAIR Data Spaces are interactive proof-of-concept showpieces, which can be used to showcase and evaluate new concepts developed in the project. The first part provided a top-level overview of the demonstrator components in a joint presentation of three demonstrators being developed within the FAIR Data Spaces project. Afterwards, the deep dive was split into three parts that individually showcased the three demonstrators. A short overview of the three sessions of the demonstrator deep dive is given in the following.
FAIR-DS Demonstrator NFDI4Biodiversity
In this session an initial demonstrator based on NFDI4Biodiversity use cases was introduced. For the NFDI4Biodiversity demonstrator, the overall goal of showing the potential when combining data from academia and industry through GAIA-X compatible clouds like the de.NBI Cloud was discussed. In particular, the presentation introduced different kinds of spatio-temporal biodiversity and geodata. Then, their combination through visual analytics was explored.
Next, a more detailed look at Geo Engine was provided. Geo Engine is a cloud-based research environment for spatio-temporal data processing that can be used for interactive analysis of geodata. First, the role of Geo Engine in the overall architecture in NFDI4Biodiversity was explained. Second, core concepts of Geo Engine such as exploratory workflows were introduced. Finally, a live demo of Geo Engine was shown featuring a variety of biodiversity data and use cases.
For user interaction, a live instance of Geo Engine hosted in the de.NBI cloud was available for participants of the session. Some datasets in the instance could be accessed through a connection to the core NFDI4Biodiversity storage. For this event, Geo Engine also provided the Normalized Difference Vegetation Index (NDVI) as monthly cloud-free aggregates for Germany.
Following the presentation and the live demo, a discussion among participants resulted in three key findings. First, a data trustee for NFDI can improve trust among different parties, which can ultimately lead to more data being shared. Second, early access to tools and storage via constantly evolving demonstrators increases visibility and improves the overall project results through agile development processes. Finally, establishing long-term financing solutions for services originating from academia is an important ongoing challenge. This demonstrator features the de.NBI cloud as an example for a community-driven infrastructure and the Geo Engine GmbH as an example for a start-up originating from research projects. Funding and promoting these types of projects is essential for creating sustainable infrastructure solutions.
Research Data Quality Assurance And Workflows
The session discussed the FAIR Data Spaces Demonstrator “FAIR Data Quality Assurance and Workflows” developed within FAIR Data Spaces together with NFDI4Ing. Together with the participants it was shown how the demonstrator uses the workflow engine provided by the source code hosting platform GitLab to analyze, transform and verify research data artifacts. Within the session research data was assumed to be collected in the form of CSV files by an individual researcher or a group of researchers who want to make use of features coming from the “social coding” paradigm to maintain their research data. The following steps were demonstrated:
- Extraction of a “Frictionless Schema” from a collection of existing CSV data
- Validation of new data based on existing schema definitions
- Assertion of data quality metrics like
- Number of missing values
- Value distribution
- Value correlations
- Generation of quality report “score cards” for research data
- Publication of research data to repositories like Zenodo
During the session the participants could interactively work with several data sets that represented different quality characteristics, like missing values, non matching data types, or malformed data. Based on the generated quality report, the participants could interactively modify a copy of these datasets and see how changes in the quality of the data set reflect in the reports.
Throughout the session the quality assurance workflows presented were run on a public-private (hybrid) cloud environment jointly provided by infrastructures at RWTH Aachen University and the Open Telekom Cloud. That environment allowed seamless scaleout for running multiple workflows at the same time while also hiding the technical complexity of the cloud based scheduling and scaling processes for the users through the workflow engine. Following the session the participants had a discussion and shared how they plan to adopt the presented data quality assurance metrics in their local environments.
Cross-Platform FAIR Data Analysis On Health Data
This session introduced an initial demonstrator of a Cross-Platform FAIR data analysis approach (Personal Health Train (PHT), NFDI4Health). The main point of this demonstrator was to represent the distributed data analysis process and the analysis of health-related data where a skin lesion data analysis is being used as a proof-of-concept showpiece. For this demonstrator, the exhibition introduced the importance of using FAIR concepts for data analysis on clinical data distributed in different medical institutions respecting data privacy and institution policies regarding data access.
In the second part of the session, further details of PHT concepts were described in more specific detail. PHT is a cross-platform data analysis that provides all the required data analysis procedures for every kind of data. An analogy to a rail network with trains and stations can be used for the main elements of the PHT ecosystem. The Train encapsulates the analysis tasks using containerisation technologies. Trains contain all prerequisites to query the data, execute the algorithm, and store the results. Stations act as data providers that maintain repositories of data. A specific Train is sequentially transmitted to every Station to analyze decentralized data. The Train performs the analytical task and calculates the results (e.g. statistics) based on the locally available data. The use case and a step-by-step preparation for the use case data to be accessed in different stations were also described during this session presentation.
During the “Bring your own code” section, A GitLab repository as a Train Registry was made available. Trains were built (GitLab CI), pushed to the PHT environment, and executed on three different test stations. The code on the PHT stations was run together with the instructors.
For further information about the PHT project, please access this link.
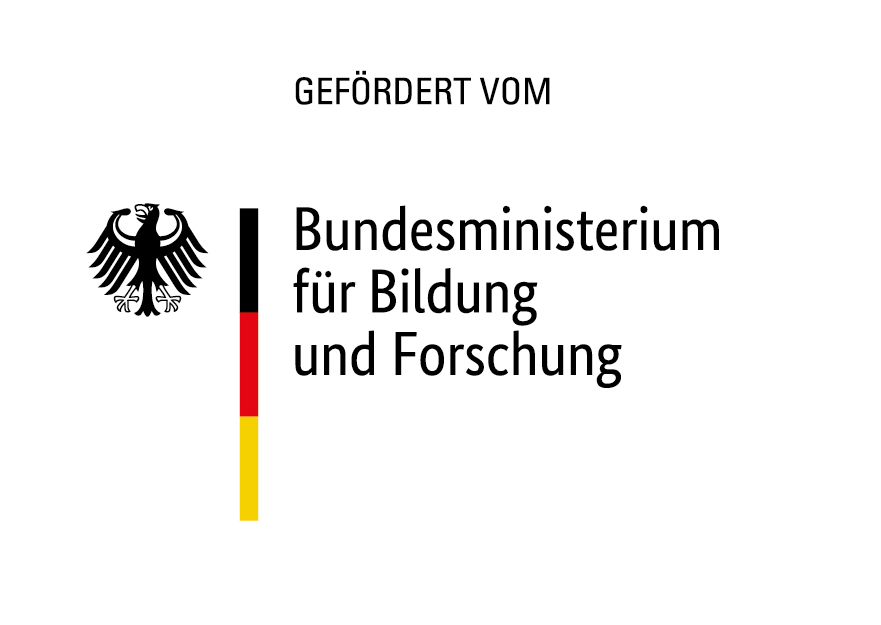